Introduction
Building a modern health tracking app means solving a complex problem early: how to integrate health data from multiple sources like Apple Health, Fitbit, and Google Fit into a consistent, usable format. Users generate fitness, wellness, and activity data across different devices and platforms, but expect your app to present a unified view without gaps or inconsistencies.
Each platform structures its data differently, from units of measurement to timestamp formats and permission models. Without proper data normalization and schema mapping, attempts to integrate health data can lead to mismatched values, missing fields, and sync errors that frustrate users and slow down development.
Here, we will explore why multi-source health data integration matters, highlight the major technical challenges developers face, and share best practices for designing a backend that keeps wearable data clean, reliable, and ready for analytics or personalization.
Key Points
- Developers must integrate health data from Apple Health, Fitbit, and Google Fit for a unified experience.
- Each platform has different APIs, data structures, and permission models.
- Aggregating data early prevents inconsistencies, syncing issues, and technical debt.
- Apple Health uses local device storage; Fitbit and Google Fit offer cloud APIs.
- Common problems include mismatched units, timestamp errors, missing data, and dynamic permissions.
- Best practices are to normalize data early, handle missing fields, optimize API calls, and store timestamps in UTC.
- Future-proof by using modular architecture, versioning schemas, and planning for new sources.
- Unified data platforms like LLIF can simplify health data aggregation and reduce engineering complexity.
Why Integrate Health Data from Multiple Sources?
Modern health tracking apps must integrate health data from multiple platforms because users rarely stick to a single device. One user might track steps with a Fitbit, log heart rate through Apple Health, and monitor sleep patterns with Google Fit. If your app pulls from only one source, it risks showing incomplete or conflicting information, which can quickly erode user trust.
Aggregation solves this problem by creating a unified and consistent dataset. When data is normalized across sources, your app can deliver better analytics, more accurate insights, and a smoother user experience. For example, combining heart rate data from different devices allows you to create continuous timelines instead of disjointed records.
Plus, aggregation reduces technical debt over time. Without a unification strategy, small inconsistencies, like variations in timestamp formats or missing fields, can cause larger issues as the app scales. Addressing these problems early helps your backend remain flexible, efficient, and easier to maintain.
Moreover, supporting multiple data providers makes your app more adaptable. It avoids locking users into a specific device ecosystem and prepares you to integrate health data from future platforms like Garmin, Oura, or Whoop without major rewrites.
Understanding the Differences Between Apple Health, Fitbit, and Google Fit APIs
Before you integrate health data from multiple platforms, it’s important to understand how Apple Health, Fitbit, and Google Fit handle their APIs. Each platform offers different data models, access methods, and permission requirements. These differences will affect how you design your integration layer.
Apple Health
Apple Health works primarily through HealthKit, which acts as a local data broker on the user’s device.
- Apps request permission to read and write specific data types, such as steps, heart rate, or sleep analysis.
- Data is stored locally on the device and is encrypted when the phone is locked.
- Sync behavior depends entirely on the device; there is no public cloud API for Apple Health.
- As a result, your app must implement a native iOS integration using HealthKit APIs.
Apple Health allows users to control permissions at a granular level. Therefore, you must plan for partial data access and dynamic permission changes over time.
Fitbit
Fitbit provides access through a cloud-based Web API.
- You must implement OAuth 2.0 authentication to connect to user accounts.
- Once authorized, your app can retrieve activity, heart rate, sleep, and other metrics through RESTful endpoints.
- Data is synced to Fitbit’s cloud servers from the user’s device before your app can access it.
Unlike Apple Health, Fitbit is platform-agnostic and can be integrated into both iOS and Android apps. However, syncing delays can occur if the user’s Fitbit device has not recently connected to the internet. Handling these delays gracefully is important for maintaining data accuracy.
Google Fit
Google Fit (being migrated to Android Health Connect) operates differently depending on whether you are targeting Android devices or using the REST API.
- On Android, Google Fit stores data locally and provides a fitness API to access it directly on the device.
- For cloud-based access, Google offers a REST API that aggregates user health data in the Google cloud.
- Permissions are handled using OAuth scopes, similar to Fitbit, but with additional complexity if users grant access to only certain datasets.
Recently, Google introduced Health Connect on Android, which aims to unify how health data is shared across apps. It acts similarly to Apple’s HealthKit but is still an evolving platform.
Because Google Fit supports both device-local and cloud-based data access, you need to decide early whether your app will pull data directly from the device, from the cloud, or from both depending on user context.
To design an effective health data integration strategy, it’s important to understand the fundamental differences between Apple Health, Fitbit, and Google Fit. The table below summarizes key aspects you will need to plan for when building your health data aggregation system.
Feature | Apple Health | Fitbit | Google Fit |
---|---|---|---|
Local Device Access | ✅ Yes (HealthKit) | ❌ No | ✅ Yes (Android devices) |
Cloud Access Available | ❌ No | ✅ Yes (required) | ✅ Yes (optional) |
Platform Dependency | ❗ iOS only | ✅ Cross-platform | ✅ Android native, cloud cross-platform |
Permission Model | ✅ Fine-grained per data type | ✅ OAuth scopes | ✅ OAuth scopes + dataset options |
Real-Time Data Sync | ✅ On device | ❗ Depends on device syncing | ✅ Device real-time, cloud delayed |
Offline Functionality | ✅ Full (local device storage) | ❗ Limited (depends on syncing) | ✅ Full (on device) |
New Initiatives | ❌ None | ❌ None | ✅ Health Connect |
Special Considerations | Must handle partial permissions and no cloud fallback | Sync delays if not connected | Must choose between local and cloud sources |
Now that we have compared the key differences between Apple Health, Fitbit, and Google Fit, it becomes clear that integrating health data is not as simple as pulling from a single API. Each platform introduces its own challenges around data access, timing, consistency, and permissions. In the next section, we will take a closer look at the most common technical problems developers face when aggregating wearable health data, and how to plan for them effectively.
Common Challenges When You Integrate Health Data from Wearables
Integrating health data from multiple wearable platforms introduces several technical challenges. Understanding these issues early can help you design a more reliable and scalable system.
Data Schema Differences
Each platform defines health metrics differently.
- Apple Health might label steps as
HKQuantityTypeIdentifierStepCount
, while Fitbit usesactivities-steps
. - Some platforms include metadata, like activity type or context, while others do not.
- Field names, data types, and nesting structures vary across APIs.
To manage these differences, create an internal unified schema that normalizes incoming data into a common format before using it in your app.
Related: LLIF offers data APIs that integrate health data from wearables under a unified schema
Units of Measurement
Not all platforms report metrics in the same units.
- Distance might be returned in meters, miles, or steps depending on the source.
- Heart rate variability might be reported in milliseconds or as a normalized score.
Always standardize units during ingestion to avoid inconsistencies in downstream analytics.
Timestamps and Time Zones
Wearable data often includes timestamps, but formats and time zone handling can vary.
- Some sources report UTC timestamps, while others use local device time.
- Missing time zone information can cause misalignment when aggregating multi-source data.
It’s best to store all timestamps internally in UTC, and track the original time zone separately if needed.
Sync Delays and Data Freshness
Not all devices sync data to the cloud immediately.
- Fitbit devices require the user’s phone to sync with the Fitbit app.
- Google Fit cloud sync can lag behind local device data depending on settings.
Design your system to handle delayed or partial data gracefully, and allow for backfilling when new data becomes available.
Dynamic User Permissions
Users can modify or revoke permissions at any time.
- Apple Health permissions can be adjusted per data type.
- Fitbit and Google Fit allow revoking OAuth scopes.
Your app must be able to detect permission changes in real time and adjust which datasets it can access without breaking user flows.
Best Practices for Health Data Aggregation in Mobile Apps
After identifying the common challenges, it’s important to design your health data integration with reliability, scalability, and flexibility in mind. Below are key best practices to follow.
Normalize Incoming Data Early
- Create an internal schema to standardize field names and data types across platforms.
For example: Map Fitbit’s activities-steps
and Apple Health’s HKQuantityTypeIdentifierStepCount
into a single internal steps
field.
{
"steps": Integer,
"distance_meters": Float,
"heart_rate_bpm": Integer
}
- Convert units (e.g., meters to miles, ms to seconds) at the point of ingestion.
- Validate all incoming data before saving it to your database.
Normalizing early prevents inconsistencies from leaking into your app’s analytics or user interface.
Handle Missing and Partial Data Gracefully
- Assume that not all fields will be available from every platform or user. For example, if Google Fit returns
null
forsleep_duration
, fallback to a default state without throwing an error. - Design your backend to tolerate missing data without causing crashes or incomplete records.
- Allow for deferred data syncing and backfilling when delayed sources eventually sync.
Flexibility in data handling improves app stability and user trust.
Implement Smart Permission Handling
- Detect permission changes dynamically, especially on iOS with HealthKit.
- Update available datasets in real time when users grant or revoke access.
- Inform users clearly if features are limited by permission restrictions.
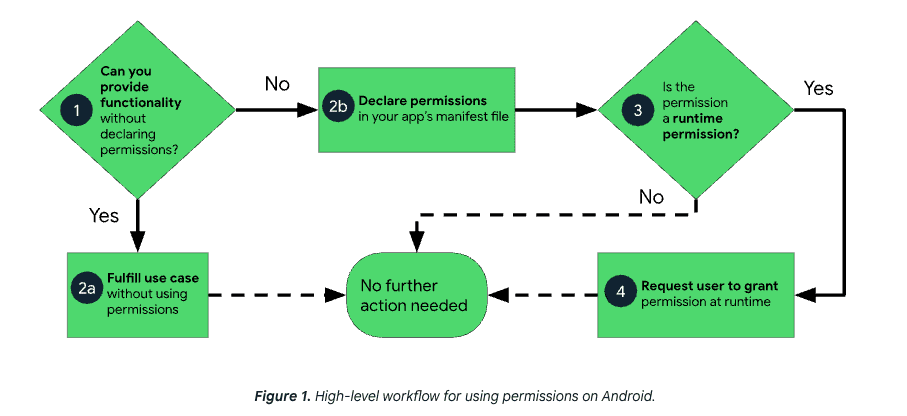
Good permission handling reduces support issues and improves the user experience.
Optimize API Usage and Sync Efficiency
- Use batch requests where possible to reduce API call volume.
- Cache recent data locally to minimize repeated API hits.
- Schedule background syncs carefully to preserve battery life and data freshness.
Efficient sync strategies help your app scale and avoid hitting platform rate limits.
Store Timestamps in a Consistent Format
- Save all timestamps in UTC to avoid local time zone conflicts.
- Track the original time zone separately if needed for display purposes.
- Normalize timestamp formats immediately upon data ingestion.
Consistent timekeeping prevents misalignment when merging multi-source health data.
Design for Future Expandability
- Build your aggregation layer to accept new sources without major code changes.
- Use modular connectors for each platform’s API.
- Plan for schema versioning to manage changes over time.
Future-proofing your architecture saves time when adding new data sources or device integrations.
Future-Proofing Your Health App for New Data Sources
Health and wearable technology are evolving quickly. To avoid major refactors later, it’s critical to integrate health data in a system with future expansion in mind.
Here are key strategies to keep your app flexible:
Use a Modular Integration Layer
- Treat each health data provider (Apple Health, Fitbit, Google Fit) as a separate module.
- Define a common interface for reading and normalizing incoming data.
- Swap or add modules without changing your core application logic.
Modular design makes it easier to add new platforms like Garmin, Oura, or Whoop later.
Version Your Internal Data Schemas
- Define schema versions for your normalized health data structures.
- Store version information with each record for easier migrations and upgrades.
- Handle schema evolution through compatibility layers rather than rewriting existing records.
Version control helps you manage inevitable changes in API formats or health metrics.
Separate Raw and Normalized Data Storage
- Keep a copy of the original, raw API responses if storage costs allow.
- Normalize and use a second, processed version for analytics and user-facing features.
- Reprocess raw data when platform updates or new normalization rules are introduced.
Storing raw data future-proofs your ability to adapt without needing to re-pull historical data.
Plan for New Contextual Data Types
- Reserve space for environmental context like pollen, air quality, or location tagging.
- Allow new metrics to be added without breaking existing queries or features.
- Extend your internal schema to support new health-related dimensions easily.
Health apps that integrate environmental and contextual data offer deeper insights and stand out from the competition.
Consider Unified Data Platforms
- Evaluate solutions that offer pre-normalized health and environmental data under a single API.
- Nonprofit platforms like LLIF aim to simplify cross-source aggregation while respecting user privacy and data ownership.
- Unified platforms can reduce engineering overhead, speed up development, and improve long-term flexibility.
Choosing the right foundation to integrate health data today can save significant time and cost as your app grows.
Conclusion
It’s not a simple task to integrate health data from Apple Health, Fitbit, and Google Fit. Each platform has its own data models, permission flows, and syncing behaviors. Without a solid aggregation strategy, your app can quickly run into problems with data consistency, user trust, and system scalability.
By normalizing incoming data early, handling partial records gracefully, and designing a modular integration layer, you can build a system that remains flexible as new devices and platforms emerge. Future-proofing your health app today will save significant rework and technical debt tomorrow.
If you are planning to expand your app’s capabilities, consider looking into unified data platforms that handle health and environmental data together. Solutions like LLIF are designed to simplify the aggregation process, reduce engineering overhead, and protect user data without commercial conflicts.
Building a reliable and scalable health app starts with making smart architectural choices. Planning ahead will help you deliver a better experience for your users while keeping your backend resilient as the health data landscape evolves.